How IoT Analytics Differs From Traditional Analytics
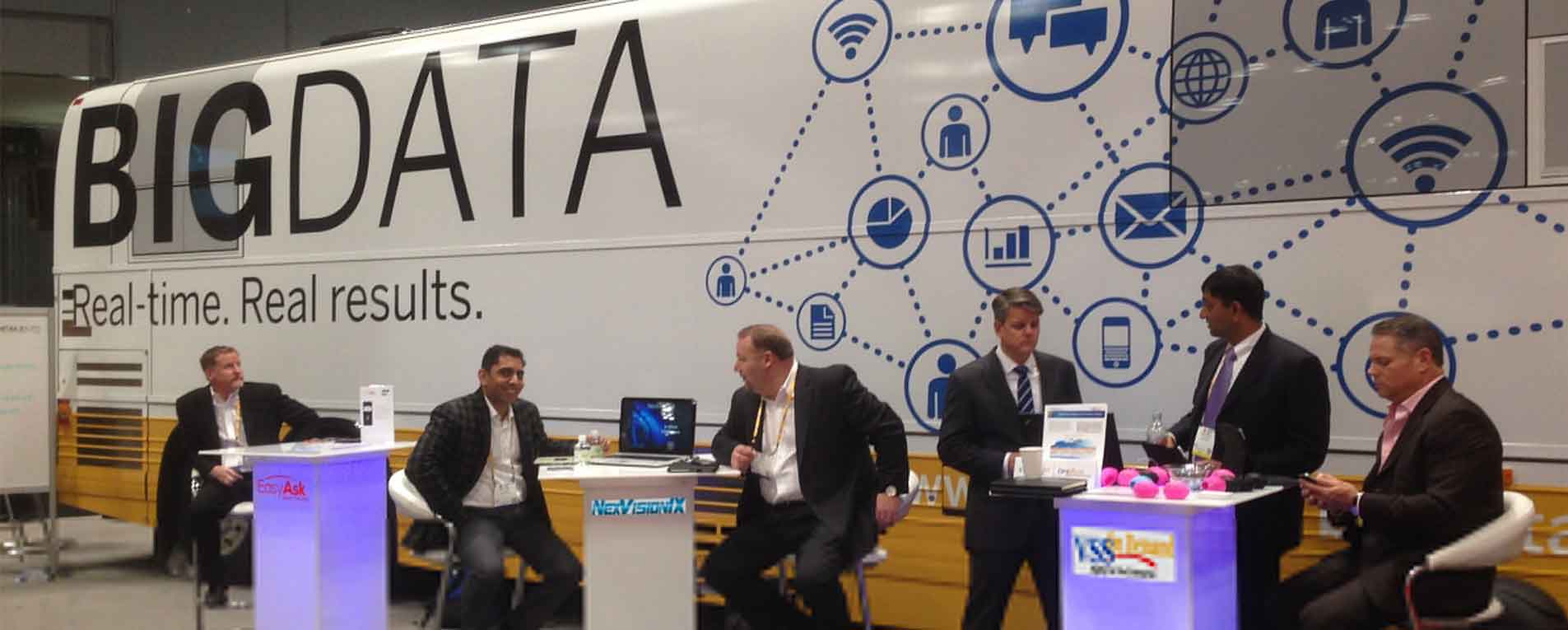
Knowing how IoT analytics differ from traditional analytics can give your company a competitive edge as we prepare for future tech advancements. The Internet of Things (IoT) market introduces the potential to drastically change many industries by connecting devices that were previously unable to network. Some examples of this technology include proximity marketing beacons located in retail stores, smartphone programmable climate-control systems in offices, and digital manufacturing gauges. While this technology remains in its infancy, the functionality and data collection possibilities require an adjustment to your organization's strategic approach.Gartner predicts that over 50 percent of new workflows, business processes and systems will leverage this technology by 2020. The thought of a work environment without IoT devices will be unthinkable for many companies. However, you face a major challenge in the present day: adapting your current analytics solutions to an IoT future.Any technology system relies on information analytics for sustained long-term success. Businesses use this data to make well-informed decisions, better forecasts and future plans. Current analytics solutions use predictive modeling and algorithms to offer sophisticated insights from your available data. Companies need human interaction and judgment to make a sound prediction based on the data presented by the analytics system. Continual adjustment, evaluation and refinement of the models and algorithms in place can improve results going forward.Organizations need a digital transformation to start using a data-driven strategy, but it’s important to remember that you don't get to rest after you pick an analytics system. You need to stay on top of the latest developments and prepare your company for market disruption. It might be a few years before the Internet of Things is in every household, but preparation should start now.Typical systems use a combination of reporting and prediction analytics, but IoT analytics requires additional real-time streaming data analysis. IoT analytics gives you a full end-to-end picture and connects the proverbial dots. Rather than having a disjointed analytics pipeline and jumping from assumption to assumption, IoT demands more data, adds complexity and requires automation and integration to function properly.
IoT's beauty comes from its ability to assemble a total data view from many sources. This information surfaces data previously overlooked or never before capable of being captured. Companies can get these unique, valuable insights due to the high volume data analysis in IoT. The data comes from streaming sensors on devices, machines and other appliances. However, the biggest challenge in IoT analytics is figuring out the optimal way to collect, manage and store this data. The optimal collection processes evolve over time as new technology comes on the market. While we can't see into the future and know exactly what data types your analytics system must tackle, you can work on a flexible system capable of growing with your organization.
As new technology introduces additional data collection methods, the need for advanced analytics rises. These modern solutions need even distribution to account for the sensor data coming from IoT devices. Businesses can't lock analytics away in the IoT devices themselves. They need the systems and servers to handle this information appropriately. Software designed to capture this data must focus on eliminating the complexity and latency associated with the enormous real-time data stream and extracting the value from it.
Many companies deal with too much IoT data to handle analytics manually. They need automation to get the most efficient and useful analysis possible. The IoT lets your company diversify its information sources and expand into new areas. Automating significant portions of this process allows you to use your resources more efficiently. IoT analytics systems only need human interaction if the system encounters an error, freeing up valuable time for only the most important tasks. Integration across business and operations systems will become the standard to create a communicative automation solution capable of high-speed big data processing.
The adoption of internet-enabled devices creates some issues with mass IoT analytics. Smartphone adoption continues to grow, but connected devices don't enjoy the same rate. Many still don't understand what IoT is or get frustrated that it doesn't work as seamlessly as expected. The market lacks common standards since the Internet of Things is a relative newcomer in the technology segment.Right now there are several technology groups collaborating on this problem, but there are still some roadblocks. Every new device uses its own software, so you have to jump through hoops to communicate with IoT hardware already on the market. A standardization system is needed to reduce complexity of the availability analytics and systematize the available data .All this sensor data needs aggregated, but every device sits in its own silo. While some manufacturers build third-party integration into their products, you don't see widespread interoperability. The real value of IoT analytics remains a dream for most industries and organizations—with the exception of manufacturing, which incorporates this technology into many business processes. Businesses need to find an analytics solution capable of scaling with the IoT market, but your organization may struggle with the data it already has. Before considering the IoT data your company is currently lacking, it’s important to dive deep into existing sources and create a strong foundation for the future. By doing the necessary groundwork now, businesses can position themselves for long-term competitive advantage by the time the IoT rules the business world.